Last Updated on 05/12/2023 by Dolly
Understanding Reinforcement Learning
Reinforcement learning, a subset of machine learning, allows machines to learn by observing actions in their environment and receiving long-term rewards. The difference here is that, unlike traditional programming, reinforcement learning allows autonomous systems to make decisions on their own and learn from the consequences, allowing them to adapt and improve over time.
Applications in Autonomous Systems
1. Autonomous Vehicles
Reinforcement learning plays a major role in training driverless vehicles. By teaching them to navigate complex road maps, interpret traffic signals, and respond in real-time situations, it increases efficiency and improves safety.
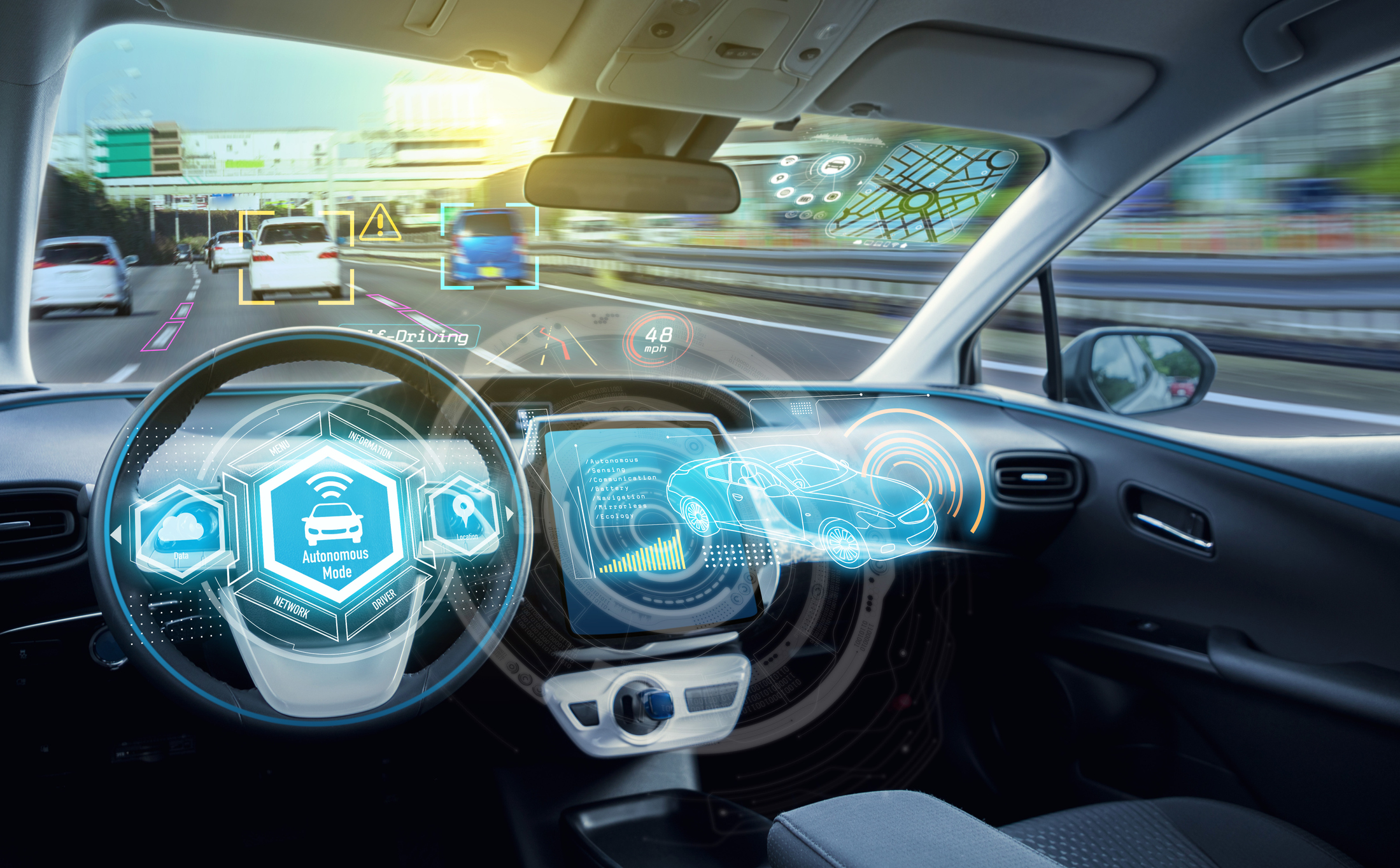
2. Robotic Manufacturing
Reinforcement learning-capable robots in production environments improve tasks like quality assurance and inspection. They acquire the ability to move precisely, which improves the accuracy of the work. Productivity consequently rises as a result.
3. Drones and Aerial Systems
Reinforcement learning enables drones to master complex flight patterns and adapt to ever-changing weather conditions. Disaster response, aerial surveys and delivery services are empowered by this technology, making it important for all aspects of life.
4. Healthcare Robotics
Reinforcement learning algorithms-based autonomous robotic systems support medical procedures, patient care, and drug delivery. The general standard of healthcare is raised as a result of these robots’ ability to precisely handle delicate procedures.
Reinforcement Learning’s Advantages for Robotics
1. Adaptability
Reinforcement learning in unpredictable and novel situations allows autonomous systems to adapt and function continuously in a variety of environments.
2. Efficiency
Robots become more efficient in their tasks by learning optimal paths and actions, helping to increase productivity and reduce operational costs.
3. Safety
Robots using reinforcement learning algorithms can make quick decisions, ensuring compliance with safety protocols even in challenging, high-risk circumstances.
4. Innovation and Future Robotics
Technologies that were previously unimaginable are now opening up new applications of robotics through the integration of reinforcement learning.
Key Takeaways
We stand at the very threshold of a new technological era involving robots and artificial intelligence. This is where reinforcement learning emerges as the driving force behind autonomous systems. The impact of reinforcement learning in autonomous systems is unparalleled and ushers in a future in which robots will be seamlessly integrated into various aspects of our lives, transforming industries and redefining the fabric of contemporary society. Reinforcement learning in autonomous systems can improve efficiency and safety as well as foster innovation.
Read More:
- Revolutionizing Machine Learning Deployment and Management
- The AI revolution in the healthcare industry
- The Importance of Explainable AI (XAI) in Understanding Intelligent Systems
- Navigating the Ethical Terrain of AI and Machine Learning step by step
- Generative Adversarial Networks (GANs) in 2023: Embracing Convenience or Unleashing Creativity?